A Product Leader's Guide to Responsible AI
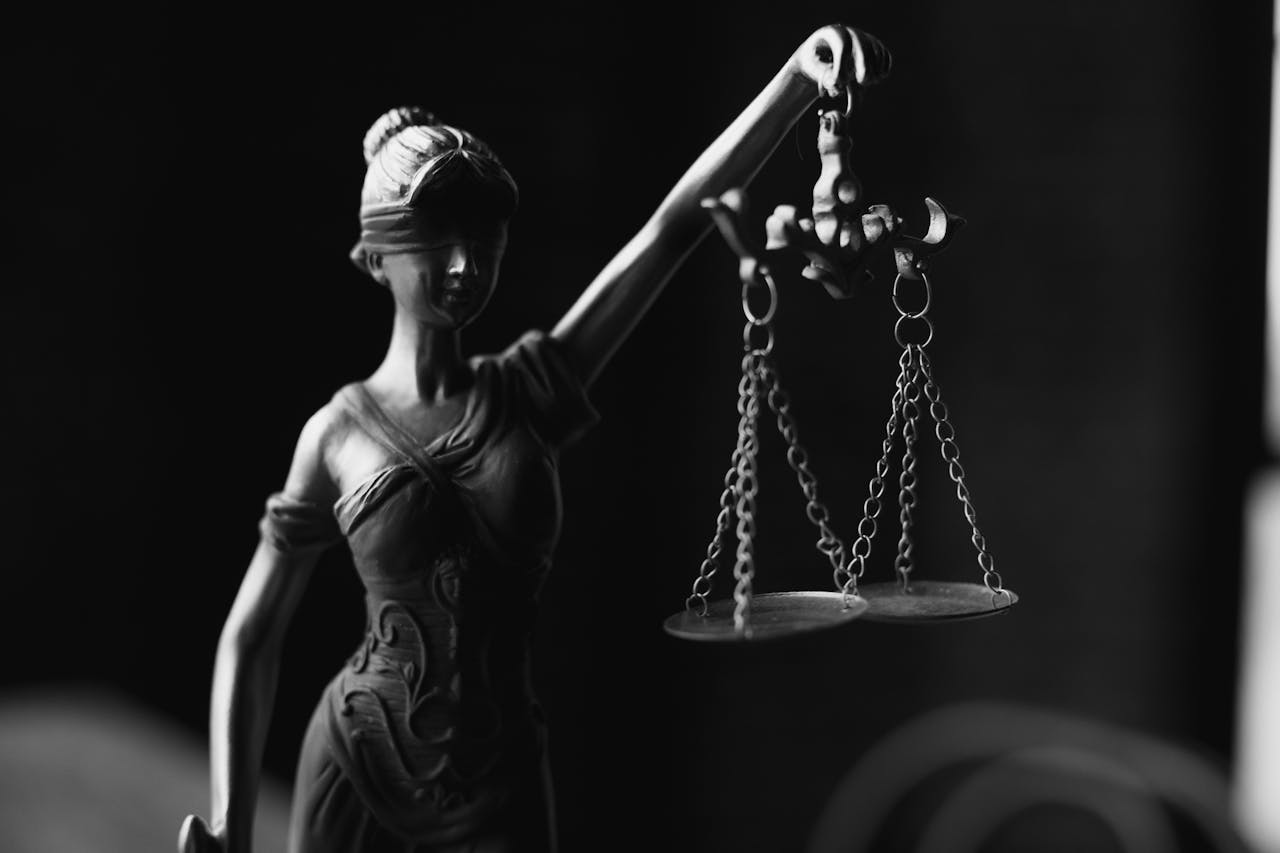
Summary
Before I begin, I must call out that thoughts on this blog are my own, and based on information publically available. —
As someone who’s been building products in the tech world for over 15 years, I’ve witnessed firsthand the incredible evolution of artificial intelligence. It’s exhilarating to see AI move from a niche technology to something that’s transforming industries and touching our lives in profound ways. But as AI becomes more powerful and pervasive, we as product leaders have a growing responsibility to ensure that we’re developing and deploying it ethically and responsibly.
Having grown up in India and now living in the heart of Silicon Valley, I’ve seen how technology can both empower and exacerbate existing inequalities. That’s why the ethical considerations of AI are not just abstract concepts to me – they’re deeply personal. They are critical to ensuring that the technology we build benefits all of humanity, not just a privileged few. We, in the tech industry, are in a unique position to shape the future of AI, and it is our responsibility to do so thoughtfully and ethically.
The Ethical Landscape of AI: Key Considerations
The ethical challenges posed by AI are multifaceted and complex. They touch upon fundamental questions about fairness, accountability, transparency, and the very nature of human-computer interaction. As we integrate AI into our products, we need to proactively address these challenges.
Here are some of the key ethical considerations that product leaders need to grapple with:
- Bias and Fairness:
- AI systems are trained on data, and if that data reflects existing societal biases, the AI will inevitably perpetuate them. This can lead to discriminatory outcomes in areas like loan applications, hiring processes, and even criminal justice. It is not just a theoretical concern but a reality that we have already witnessed in several real-world applications.
- As product leaders, we need to be vigilant about identifying and mitigating bias in our AI systems. This involves carefully curating our training data, using techniques like data augmentation, and regularly auditing our models for fairness. We also need to be transparent about the limitations of our AI systems and their potential for bias.
- Transparency and Explainability:
- Many AI systems, particularly deep learning models, are often described as “black boxes.” It can be difficult to understand how they arrive at a particular decision or prediction. This lack of transparency can erode trust and make it difficult to identify and correct errors or biases. It also raises concerns about accountability.
- We need to strive for greater transparency and explainability in our AI systems. This might involve using more interpretable models, developing tools for visualizing and understanding AI decision-making, and providing clear explanations to users about how AI is being used in our products. Explainability is not just a technical challenge but also a design challenge.
- Accountability and Governance:
- As AI systems become more autonomous, it raises questions about who is responsible when things go wrong. Is it the developer, the user, or the AI itself? Establishing clear lines of accountability is crucial for building trust and ensuring responsible use of AI. It is also important to have mechanisms in place for redressal in case of errors or harm.
- We need to establish robust governance frameworks for AI development and deployment. This might involve creating internal ethics boards, developing clear guidelines for AI development, and establishing processes for auditing and monitoring AI systems. It is also important to engage with external stakeholders, such as ethicists, policymakers, and civil society organizations.
- Privacy and Security:
- AI systems often rely on vast amounts of personal data, raising concerns about privacy and security. Data breaches or misuse of data can have serious consequences for individuals and society. It is also important to consider the potential for AI to be used for surveillance and the implications for individual freedoms.
- We need to prioritize data privacy and security in the design and development of our AI systems. This involves implementing robust security measures, using techniques like differential privacy and federated learning, and being transparent with users about how their data is being collected, used, and protected. Data minimization and purpose limitation should be guiding principles.
- Job Displacement and Economic Impact:
- AI-driven automation has the potential to displace workers in certain industries, leading to job losses and economic disruption. While AI will also create new jobs, the transition may be challenging for many workers. This raises questions about the social responsibility of tech companies and the need for policies to support workers and ensure a just transition.
- As product leaders, we need to consider the broader economic and social impacts of our AI products. This might involve investing in retraining programs, partnering with educational institutions, and advocating for policies that support workers and promote a more equitable distribution of the benefits of AI. We should also explore ways in which AI can be used to create new economic opportunities and empower workers.
Building a Framework for Responsible AI Development
Navigating these ethical challenges requires a proactive and systematic approach. We need to build frameworks for responsible AI development that guide our product decisions and ensure that ethical considerations are integrated into every stage of the product lifecycle.
Here are some key elements of such a framework:
- Establish Ethical Principles:
- Start by defining a set of ethical principles that will guide your AI development efforts. These principles should reflect your company’s values and your commitment to responsible AI. They could include principles like fairness, transparency, accountability, privacy, and human well-being. These principles should be widely disseminated and understood within the organization.
- Diverse and Inclusive Teams:
- Building ethical AI requires diverse perspectives and expertise. Ensure that your product and engineering teams are diverse and inclusive, representing a wide range of backgrounds, experiences, and viewpoints. This will help you anticipate and address potential ethical issues that might be overlooked by a more homogenous team. Diversity is not just a matter of social responsibility but also a source of innovation and better decision-making.
- Ethical Risk Assessments:
- Conduct thorough ethical risk assessments for your AI products, identifying potential risks related to bias, fairness, transparency, privacy, and other ethical considerations. This should be an ongoing process, revisited at different stages of the product development lifecycle. It is also important to involve external stakeholders in the risk assessment process to gain diverse perspectives.
- Data Governance and Auditing:
- Implement robust data governance policies to ensure that the data used to train your AI models is collected, stored, and used responsibly. Regularly audit your training data for bias and fairness. Establish clear guidelines for data retention, access, and deletion. Transparency and accountability should be built into the data governance process.
- Human-in-the-Loop Systems:
- Design AI systems that keep humans in the loop, especially for critical decision-making processes. This allows for human oversight and intervention when necessary, reducing the risk of errors or unintended consequences. Human oversight can also help build trust and provide a mechanism for recourse in case of errors.
- Continuous Monitoring and Evaluation:
- Monitor your AI systems after deployment to identify and address any ethical issues that may arise. Establish feedback mechanisms to gather input from users and stakeholders. Be prepared to iterate and improve your AI systems based on real-world feedback and changing societal expectations. Continuous improvement should be a core principle of responsible AI development.
- Collaboration and Knowledge Sharing:
- Engage with the broader AI ethics community, including researchers, ethicists, policymakers, and civil society organizations. Share best practices, lessons learned, and challenges encountered. Participate in industry-wide initiatives to develop ethical guidelines and standards for AI. Collaboration is essential for advancing the field of AI ethics and ensuring that AI benefits all of humanity.
The Path Forward: Embracing Ethical AI
The development of ethical AI is not just a technical challenge; it’s a societal imperative. It requires a fundamental shift in how we think about product development, a shift that puts ethical considerations at the heart of everything we do. As product leaders, we have a unique opportunity and responsibility to shape the future of AI in a way that benefits all of humanity.
This journey will undoubtedly be complex and challenging. There will be difficult trade-offs and unforeseen obstacles. But I’m optimistic that we can rise to the occasion. By embracing a proactive, collaborative, and human-centered approach to AI development, we can build a future where AI is a force for good in the world, a future where technology empowers us to create a more just, equitable, and sustainable society. The potential benefits of AI are immense, and it is our responsibility to ensure that these benefits are realized in a way that aligns with our values and aspirations as a society.
On a personal note, I find the ongoing conversations about AI ethics to be both stimulating and essential. They remind me that we’re not just building products; we’re shaping the future.
And as someone who came to this country with hopes and dreams, I feel a deep sense of responsibility to ensure that this future is one we can all be proud of. I am also encouraged by the growing awareness and commitment to ethical AI within the tech industry and beyond.
If you’d like to talk more, feel free to connect with me.