Trust in the Machine: Building a Future Where We Confidently Embrace AI
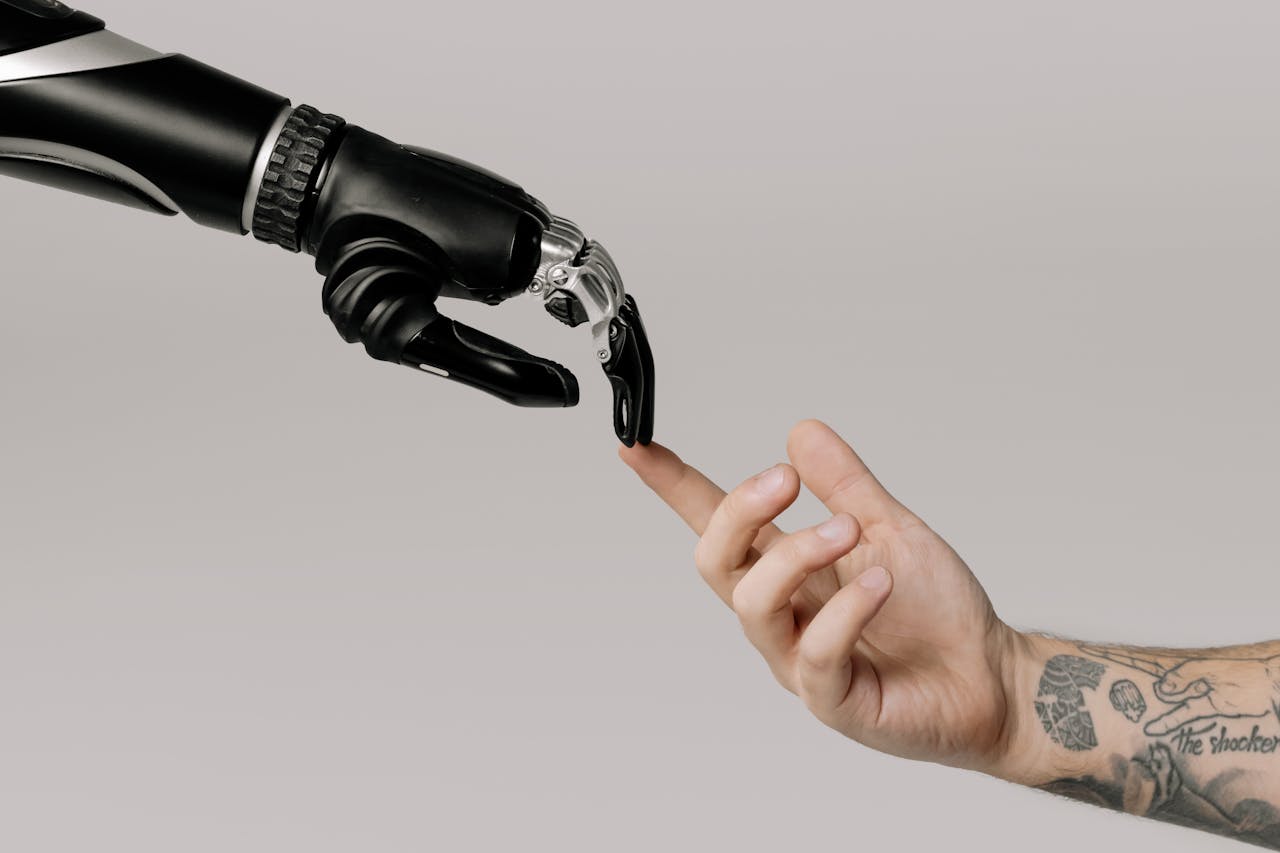
Summary
Before I begin, I must call out that thoughts on this blog are my own, and based on information publically available. —
As AI becomes increasingly woven into the fabric of our lives, we must but wonder: can we trust it?
The engineers, entrepreneurs and the product managers of the world are constantly pushing the boundaries of what’s possible with technology.
As a product leader and a thoughtful consumer, I’ve learned that innovation without trust is like a ship without a rudder – it might move fast, but it’s unlikely to reach its intended destination. This is particularly true for AI, a technology with immense potential to improve our lives but also with the capacity to erode trust if not developed and deployed responsibly.
Having grown up in India, I witnessed firsthand the power of technology to bridge divides and create opportunities. But I also saw how a lack of access and understanding can lead to mistrust and exclusion. That’s why the issue of trust in AI is so important to me. It’s not just about building sophisticated algorithms; it’s about building systems that are worthy of the public’s trust, systems that are transparent, explainable, and ultimately, under human control. Without trust, AI cannot achieve its full potential to benefit humanity.
Why Trust in AI Matters
Trust is the foundation of any successful relationship, and our relationship with AI is no exception. If users don’t trust AI systems, they won’t use them, plain and simple. And if AI systems aren’t used, they can’t deliver on their promise to improve our lives. It is a virtuous cycle – trust leads to adoption, which in turn leads to improvement and further trust.
Here’s why trust in AI is so crucial:
- Adoption and Engagement: Users are more likely to adopt and engage with AI products they trust. Whether it’s a self-driving car, a medical diagnosis system, or a personalized recommendation engine, users need to feel confident that the AI is working in their best interests. Without trust, users may be hesitant to rely on AI, even if it offers significant benefits.
- Safety and Well-being: In many applications, AI systems have a direct impact on our safety and well-being. We need to trust that self-driving cars will make safe decisions, that medical AI will provide accurate diagnoses, and that financial AI will manage our money responsibly. Trust is essential for ensuring that AI systems are used safely and effectively in critical domains.
- Fairness and Equity: As discussed in earlier posts, AI systems can perpetuate and even amplify existing societal biases. Building trust requires ensuring that AI systems are fair and equitable, and that they don’t discriminate against certain groups of people. Trust is essential for ensuring that AI benefits all members of society, not just a privileged few.
- Social Acceptance: For AI to be truly integrated into society, it needs to be accepted by the public. This requires building trust not just in individual AI systems but in the broader project of AI development. Trust is essential for fostering public support for AI research and development, and for ensuring that AI is used for the benefit of humanity.
Building Blocks of Trust: Transparency, Explainability, and User Control
So, how do we build AI systems that are worthy of our trust? I believe there are three key building blocks:
-
Transparency:
- Data Transparency: Users should have a clear understanding of what data is being collected about them, how it’s being used to train AI models, and with whom it’s being shared. Data collection and usage practices should be transparent and easy to understand, even for non-technical users.
- Algorithmic Transparency: While the inner workings of complex AI models may be difficult to fully comprehend, users should have some insight into the factors that influence an AI’s decisions. This might involve providing high-level explanations of how the AI works or highlighting the key factors that contributed to a specific outcome.
- Model Limitations: It’s crucial to be transparent about the limitations of AI systems. No AI is perfect, and users need to understand what the AI can and can’t do. This helps manage expectations and prevent overreliance on AI. Overstating the capabilities of AI can erode trust in the long run.
-
Explainability:
- Decision Rationale: Whenever possible, AI systems should provide explanations for their decisions or recommendations. This helps users understand why the AI made a particular choice and whether they should trust it. For example, a medical AI that diagnoses a disease should also provide an explanation of the factors that led to that diagnosis.
- Interpretable Models: In some cases, it may be possible to use AI models that are inherently more interpretable than others. For example, decision trees are generally easier to understand than deep neural networks. Choosing the right model for the task is crucial for ensuring explainability.
- Visualization Tools: Visualizations can be a powerful way to make complex AI systems more understandable. For example, visualizing the decision-making process of a neural network can help users gain insights into how the AI works. These tools can be especially helpful for developers and researchers.
-
User Control:
- Customization and Personalization: Users should have the ability to customize AI systems to their individual needs and preferences. This might involve adjusting the sensitivity of a recommendation engine, setting privacy preferences, or choosing the level of automation in a self-driving car. Customization empowers users and builds trust.
- Override and Intervention: Users should always have the ability to override or intervene in the decisions made by AI systems. This is particularly important in safety-critical applications. For example, a driver should always be able to take control of a self-driving car. This ensures that humans remain in control of the technology.
- Feedback Mechanisms: AI systems should provide mechanisms for users to provide feedback on the AI’s performance, including reporting errors or biases. This feedback should be used to improve the AI system over time. Feedback loops are essential for continuous improvement and building trust.
- Right to Opt-Out: Users should have the right to opt-out of using AI systems or specific AI features altogether. This is especially important for applications that involve sensitive personal data or have significant ethical implications. Respecting user choice is crucial for building trust.
A Product Leader’s Role in Building Trustworthy AI
As product leaders, we have a critical role to play in building trust in AI. It’s our responsibility to ensure that ethical considerations are at the forefront of our product development process. We should be champions for transparency, explainability, and user control, and we should work to create a culture of responsible AI development within our organizations.
Here are some practical steps we can take:
- Integrate Ethics into the Product Development Lifecycle: Ethical considerations should be integrated into every stage of the product development process, from ideation to design, development, testing, and deployment. This is not just an afterthought but a core part of the process.
- Establish Clear Ethical Guidelines: Develop clear ethical guidelines for AI development within your organization, and ensure that they are understood and followed by everyone involved in the product development process. These guidelines should be regularly reviewed and updated as the field of AI evolves.
- Foster a Culture of Responsibility: Create a culture where ethical considerations are openly discussed and debated, and where everyone feels empowered to raise concerns. Encourage a sense of ownership and accountability for the ethical implications of our work.
- Invest in Research and Development: Invest in research and development on topics like AI safety, fairness, transparency, and explainability. Support the development of tools and techniques that can help build more trustworthy AI systems. This investment is crucial for advancing the field of AI ethics and ensuring the long-term success of AI.
- Engage with External Stakeholders: Engage with ethicists, policymakers, and other stakeholders to gain a broader perspective on the ethical implications of AI. Participate in industry-wide initiatives to develop ethical standards and best practices. Collaboration is essential for addressing the complex ethical challenges of AI.
- Educate and Empower Users: Be proactive about educating users on how AI systems work, their limitations, and their rights. Empower users to make informed choices about their interactions with AI and to provide feedback on their experiences. Building trust requires open and honest communication with users.
The Future of Trust in AI
Building trust in AI is an ongoing journey, not a destination. It will require continuous effort, adaptation, and collaboration. But I’m optimistic that we can create a future where AI is a trusted and beneficial force in our lives. It requires a collective effort from the entire tech industry, as well as policymakers, researchers, and the public.
The stakes are high, but the potential rewards are even higher. By prioritizing trust, we can unlock the full potential of AI to improve our lives, address global challenges, and create a more equitable and prosperous future for all. I am confident that we can rise to the challenge and build a future where we can confidently embrace AI as a partner in progress. This is not just a technological challenge but also a social and moral imperative.